Our aim is to bridge the scale gap between site observations (≤1 km) and climate models (10-100 km). The framework uses drones with sensors to observe greenhouse gas concentrations and other meteorological parameters in the region of interest. Through Bayesian data assimilation, the drone observations are fused with a model to obtain an estimation of the unobserved surface greenhouse gas fluxes. The high temporal variability of the atmosphere challenges the quantification of surface fluxes and brings out the need for intelligent sampling strategies. We use reinforcement learning to optimize the positions of the drone observations, and consequently increase the accuracy of the inferred surface greenhouse gas flux maps. The framework is developed as a single-agent system as well as a multi-agent system: a drone swarm. The objective of the resulting flux maps is to facilitate climate model calibration and validation, and ultimately reduce uncertainties in climate projections.
Swarm intelligence for observing systems in climate science - developing a reinforcement learning framework for surface flux mapping with drones
Accurate mapping of surface greenhouse gas fluxes is necessary for the validation and calibration of climate models. In this project, we develop a novel framework using drone observations and machine learning to estimate greenhouse gas fluxes at a regional scale.
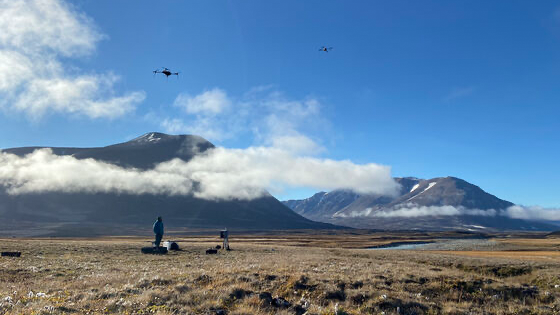
Published July 6, 2023 2:15 PM
- Last modified Oct. 23, 2023 1:34 PM